Listen to this blog
Why AI Accuracy is a Non-Negotiable
Bang! In 2023, suddenly the world was alight with chatter about the paradigm shifting new technology called ChatGPT. It was near magic in its abilities. Answers to just about any question you could imagine. And the answers were always so direct, authoritative, and convincing. Yet, on deeper inspection, AI accuracy is not what you should call it. In fact, a study from Oxford University found that large language model (LLM) AI technology (ChatGPT 4o specifically) hallucinates at least 58% of the time. Does that sound too high? Not so, as OpenAI (developer of ChatGPT), confirmed that the LLM only gets it right 42.7% of the time (supporting the 57.3% error observation).
Odd, that in a world where technical accuracy is paramount, that businesses and industries could rely on LLM based AI solutions that can go ‘off the rails’ so often. Yet, enterprises are increasingly adopting AI to streamline operations, enhance decision-making, and improve customer interactions. Fortunately, “AI Accuracy” is emerging as a concept with increasing importance. And companies are right to be concerned and ask more AI vendors about this topic.
What Does “AI Accuracy” Mean?
AI accuracy refers to the ability of an artificial intelligence system to produce results that align with the intended outcomes. This based on the quality of its underlying data, algorithms, and implementation. In applications like predictive analytics, AI agents, or automated decision-making, accuracy determines the effectiveness and reliability of the output. Accurate systems are vital for industries where errors can lead to significant financial, reputational, or even life-threatening consequences.
The Cost of Inaccuracy
AI systems are only as good as the results they produce. Inaccurate outputs can result in cascading failures. Some of the most pressing consequences of AI inaccuracy include:
-
- Reputational Damage:
In customer-facing applications, such as chatbots, inaccuracies can erode customer trust. Think of an AI virtual agent providing erroneous information about financial services. This will tarnish a company’s brand and erode the trust of that customer. Worse yet, if the customer posts the experience on social channels, it can substantially harm the brand’s reputation. It may even be the cause of legal lawsuits against the firm. - Operational Inefficiencies:
Inaccuracies can misdirect resources, driving up costs. For example, a predictive maintenance system flagging the wrong equipment for repair can disrupt workflows and inflate maintenance budgets unnecessarily. - Regulatory and Legal Risks:
For industries like finance and healthcare, regulatory compliance hinges on accurate data. Reliable decision making requires the correct information, every single time. Errors in these high-stakes fields can lead to legal liabilities and regulatory penalties. - Loss of Human Trust:
Inaccurate AI undermines the confidence of employees, customers, and stakeholders. Trust is hard to build and easy to lose, making accuracy a linchpin for sustainable AI adoption.
- Reputational Damage:
Why AI Accuracy Matters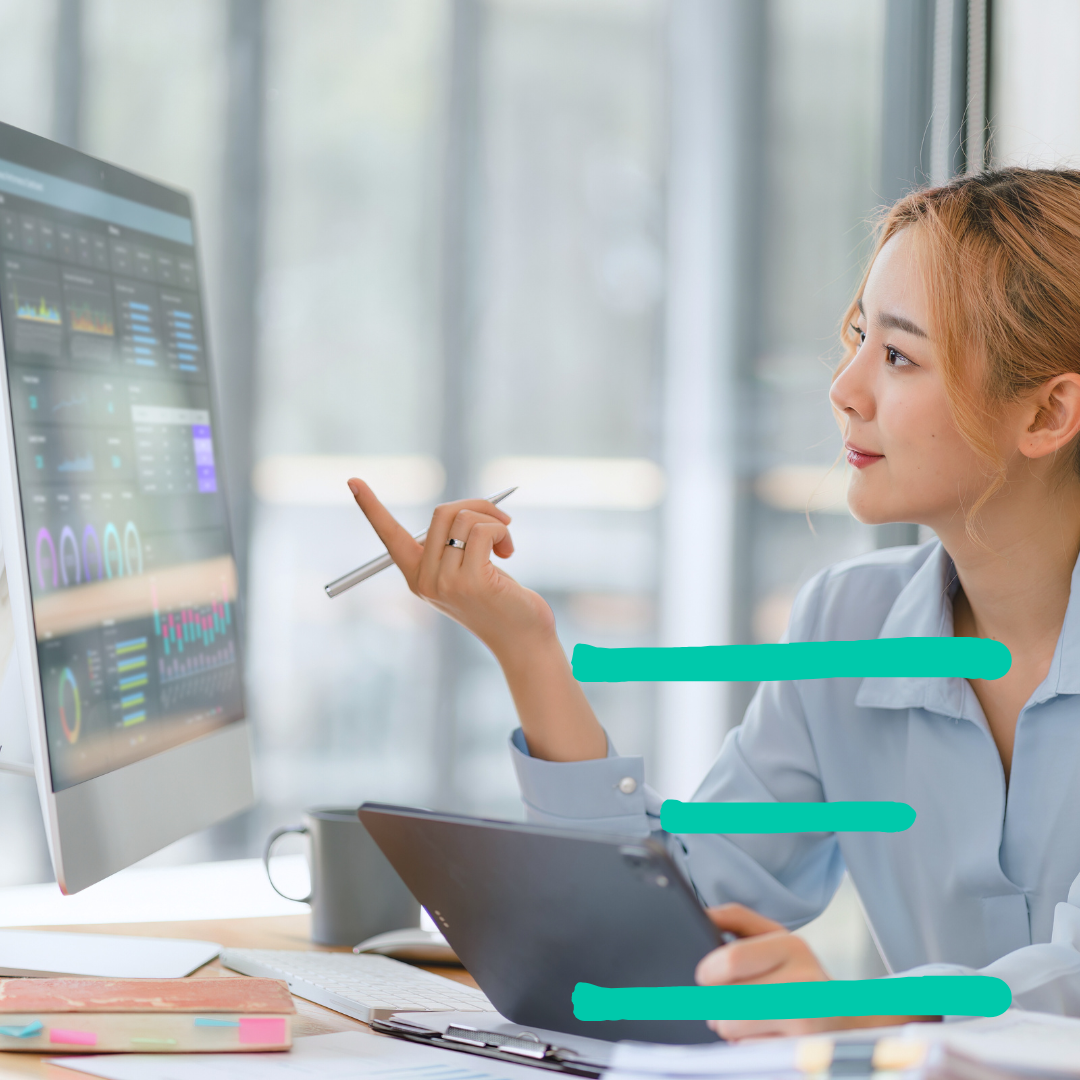
The need for accurate AI spans across various sectors such as:
-
- Healthcare:
AI tools used for disease diagnosis or treatment recommendations must operate with near-perfect precision. A misdiagnosis or inappropriate treatment suggestion puts lives at risk. - Finance:
Fraud detection, credit scoring, and investment analysis demand accurate systems. These safeguard financial assets and customer data. Equally important is when customer inquiries need to be answered. They have to be answered correctly, every single time. - Customer Service:
Virtual agents and chatbots must provide accurate responses to ensure customer satisfaction and operational efficiency. Consistently is another high importance issue. When a customer gets an answer, should they ask again, the answer had better be the same as the last time, without variation. Otherwise, trust is eroded.
- Healthcare:
Building AI Accuracy: Strategies and Best Practices
Given the stakes, how can organizations ensure their AI systems achieve and maintain high accuracy?
-
- Adopt Graph-Based AI Architectures
Unlike probabilistic Large Language Models (LLMs), graph-based AI systems inherently provide higher levels of accuracy by relying on deterministic processes. Graph AI structures data into interconnected nodes. These enable better contextual understandings. They also eliminate the risks of hallucinations. - Human-in-the-Loop Governance
Human oversight is a critical layer to ensuring accuracy. Using human validation during the AI development and deployment stages, organizations mitigate the risks of errors before they reach end-users. Humans in the loop, also ensure biased answers, racial slurs, bigotry and other nasty consequences are spotted, so they never get conveyed to a user, prospect, or customer. - Retrieval-Augmented Generation (RAG):
RAG-based systems improve accuracy by using domain-specific data to generate answers. By drawing directly from verified sources, RAG ensures that AI outputs align with enterprise trusted sources.
- Adopt Graph-Based AI Architectures
Case Study: AI Accuracy in Action
One of the notable examples of prioritizing AI accuracy is the implementation of graph-based virtual agents at Canadore College. By employing a knowledge graph-driven AI system, the institution was able to deploy a virtual assistant capable of providing students and staff with accurate, and relevant responses. The deterministic nature of the graph AI ensured that only verified, contextual data was used to generate replies. It minimized errors and improved trust in the system. Beyond that, it also offloaded the menial work of answering mundane and repetitive student, staff, and visitor questions.
Unlike LLM-driven models, which can hallucinate or produce incorrect outputs, the Canadore College solution focuses on high accuracy. It emphasizes the importance of “getting it right” over providing generalized answers. Not only does this enhance user experience, but it also demonstrates the potential for graph-based AI in critical environments.
The Future Imperative
As organizations scale AI initiatives, accuracy will remain a core determinant of success. While creative AI models like LLMs offer flexibility and innovation, they are often unsuitable for applications that need precision and trust. Graph AI, with its structured, deterministic approach, represents a viable alternative for businesses that just can’t afford inaccuracies.
Moreover, as regulatory frameworks evolve to address AI accountability, organizations must proactively adopt technologies and practices that prioritize accuracy. By integrating governance models, leveraging human oversight, and employing advanced AI techniques like RAG, enterprises can position themselves as leaders in the responsible AI space.
Final Thoughts
Accuracy is not a “nice-to-have” feature for AI solutions. Rather, it is a non-negotiable requirement. Businesses that fail to prioritize accuracy risk degrading their own abilities to deliver results. They risk operational inefficiencies, customer dissatisfaction, and regulatory challenges. With a shift towards accurate, graph-based AI solutions, there is a shift in prioritizing getting the right answers to the people who need it.
As the market continues to grow, enterprises must answer a powerful question: Can your AI be trusted to deliver the right answers, every time?